Despite the fact that AI is all code and calculations, it can in any case convey inclination and oppress individuals.
Man-made consciousness abilities are extending dramatically, with AI currently being used in ventures from promoting to clinical examination. The utilization of AI in more delicate regions like facial acknowledgment programming, recruiting calculations, and medical care arrangement, have accelerated discussion about inclination and reasonableness.
Inclination is a well-informed feature of human brain science. Exploration consistently uncovered our oblivious inclinations and biases, and now we see AI mirror a portion of these predispositions in their calculations.
Things being what they are, how does man-made brainpower become one-sided? Furthermore, what difference does this make?
How Does AI Become Biased?
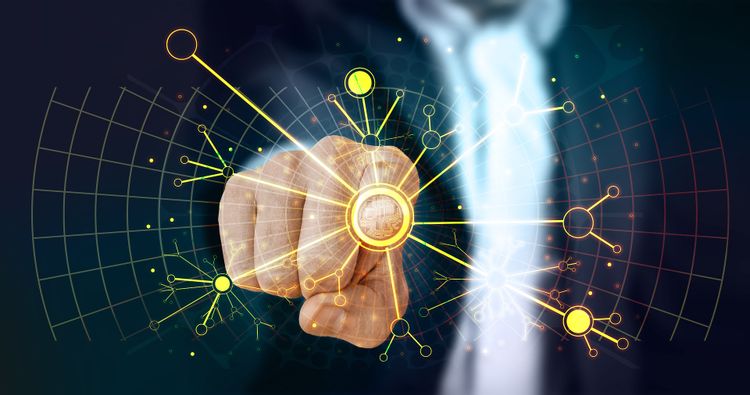
For straightforwardness, in this article, we’ll allude to AI and profound learning calculations as AI calculations or frameworks.
Scientists and designers can bring inclination into AI frameworks twoly.
Right off the bat, the intellectual predispositions of analysts can be installed into AI calculations coincidentally. Intellectual inclinations are oblivious human insights that can influence how individuals decide. This turns into a critical issue when the inclinations are with respect to individuals or gatherings of individuals and can hurt those individuals.
These predispositions can be presented straightforwardly yet inadvertently, or scientists may prepare the AI on datasets that were themselves influenced by inclination. For example, a facial acknowledgment AI could be prepared utilizing a dataset that just incorporates fair looking countenances. For this situation, the AI will perform better when managing fair looking countenances than dim. This type of AI inclination is known as a negative heritage.
Also, predispositions can emerge when the AI is prepared on fragmented datasets. For example, if an AI is prepared on a dataset that just incorporates PC researchers, it won’t address the whole populace. This prompts calculations that neglect to give precise forecasts.
Instances of Real World AI Bias
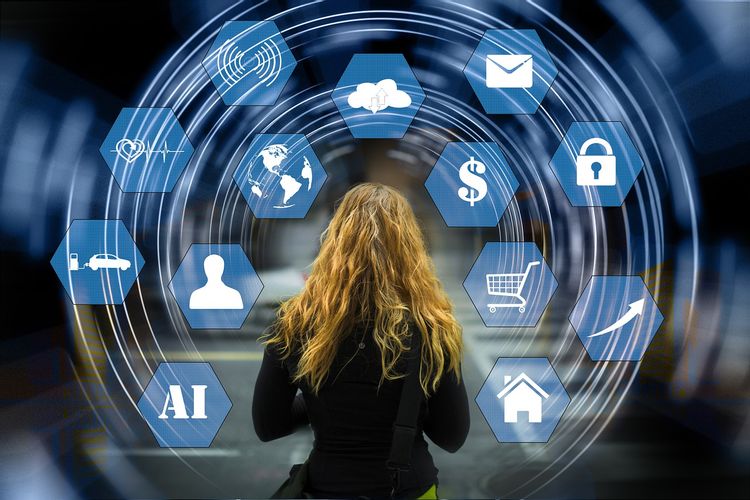
There have been different later, all around revealed instances of AI predisposition that show the risk of permitting these inclinations to sneak in.
US-Based Healthcare Prioritization
In 2019, an AI calculation was intended to help medical clinics and insurance agencies figure out which patients would profit most from certain medical services programs. In view of an information base of around 200 million individuals, the calculation supported white patients over dark patients.
It was resolved that this was a result of a flawed suspicion in the calculation with respect to shifting medical care costs among highly contrasting individuals, and the inclination was at last diminished by 80%.
COMPAS
The Correctional Offender Management Profiling for Alternative Sanctions, or COMPAS, was an AI calculation intended to anticipate whether specific individuals would re-affront. The calculation created twofold the bogus positives for dark wrongdoers contrasted and white guilty parties. For this situation, both the dataset and model were imperfect, presenting substantial inclination.
Amazon
The recruiting calculation that Amazon uses to decide the appropriateness of candidates was found in 2015 to support men over ladies intensely. This was on the grounds that the dataset only contained men and their resumes since most Amazon workers are male.
Instructions to Stop AI Bias
Artificial intelligence is as of now changing the manner in which we work across each industry. Having one-sided frameworks controlling delicate dynamic cycles is not exactly attractive. Best case scenario, it diminishes the nature of AI-based examination. To say the least, it effectively harms minority gatherings.
There are instances of AI calculations previously being utilized to help human dynamic by lessening the effect of human psychological predispositions. Due to how AI calculations are prepared, they can be more precise and less one-sided than people similarly situated, bringing about more attractive dynamic.
In any case, as we’ve appeared, the inverse is additionally obvious. The dangers of permitting human inclinations to be cooked into and enhanced by AI may exceed a portion of the potential advantages.
Toward the day’s end, AI is just pretty much as great as the information that it’s prepared with. Creating unprejudiced calculations requires broad and careful pre-examination of datasets, guaranteeing that information is liberated from understood inclinations. This is harder than it sounds on the grounds that so many of our inclinations are oblivious and regularly difficult to distinguish.
Difficulties in Preventing AI Bias
In creating AI frameworks, each progression should be evaluated for its capability to implant inclination into the calculation. One of the main considerations in forestalling inclination is guaranteeing that reasonableness, instead of predisposition, gets “cooked into” the calculation.
Characterizing Fairness
Decency is an idea that is generally hard to characterize. Indeed, it’s a discussion that is never arrived at an agreement. To make things considerably more troublesome, when creating AI frameworks, the idea of decency must be characterized numerically.
For example, as far as the Amazon employing calculation, would decency resemble an ideal 50/50 split of male to female specialists? Or then again an alternate extent?
Deciding the Function
The initial phase in AI advancement is to decide precisely the thing it will accomplish. In the event that utilizing the COMPAS model, the calculation would anticipate the probability of crooks reoffending. At that point, clear information inputs should be resolved to empower the calculation to work. This may require characterizing significant factors, for example, the quantity of past offenses or the kind of offenses submitted.
Characterizing these factors appropriately is a troublesome however significant advance in guaranteeing the reasonableness of the calculation.
Making the Dataset
As we’ve covered, a significant reason for AI predisposition is deficient, non-delegate, or one-sided information. Like the instance of facial acknowledgment AI, the information should be altogether checked for predispositions, propriety, and fulfillment before the AI cycle.
Picking Attributes
In the calculations, certain traits can be thought of or not. Characteristics can incorporate sexual orientation, race, or training—essentially anything that might be imperative to the calculation’s errand. Contingent upon which credits are picked, the prescient precision and inclination of the calculation can be seriously affected. The issue is that it’s exceptionally hard to quantify how one-sided a calculation is.
Man-made intelligence Bias Isn’t Here to Stay
Man-made intelligence inclination happens when calculations make one-sided or wrong forecasts due to one-sided inputs. It happens when one-sided or deficient information is reflected or intensified during the turn of events and preparing of the calculation.
Fortunately with financing for AI research increasing, we’re probably going to see new techniques for decreasing and in any event, killing AI inclination.